Overview of Statistics
Most people cringe at the word statistics and we understand why. Math and statistics can be complex and difficult to understand. There are various meanings for the word statistics, which adds to its confusion. With a mixture of math and logic, statistics is a branch of mathematics that is concerned with collection, analysis and interpretation of data 1. Data are scores and values that we obtain from measuring the outcomes (dependent variables) of interest in a study. Collecting data is only one piece of the puzzle, if researchers don’t know what to do with the data and how to properly describe the data, then the findings may seem underwhelming. Statistics are a way of describing data characteristics and examining the relationships between variables, this allows for greater objectivity when interpreting research and drawing conclusions. This section provides a simple overview of some common and basic statistical concepts that you will encounter throughout exercise and nutrition research. Again, this is a brief section and doesn’t even scratch the surface of the broader and more complex statistical methods that exist. Statistics operate under a number of assumptions and rules, if these are violated, they can misrepresent the data. Statistics is not our area of expertise and it’s important to realize that if you don’t fully understand statistics they can be misused to deceive people into believing the data is more appealing than it actually may be.
Percent Change
Very simply, this is the change between two values expressed as a percentage. You have to be careful with percentage change because it can sometimes appear to be a greater change than it actually is. That’s why you also want the raw or true values. For example, if a study is looking at leptin changes and they have a baseline value of 0.3ng/mL and a post-test value of 1.0ng/mL, the absolute change is 0.7ng/mL, but the percentage change is 233% [(1 - 0.3) / (0.3 x 100)]. While this change is minimal and may not be meaningful, the percentage change can make it appear as if it’s a big deal.
Central Tendency
The mean is probably one of the most commonly understood mathematical terms. The mean describes the average value of a group of numbers. In statistics, the mean is a measure of central tendency, which represents a central or balance point within a set of data 2. The mode and median are similar to the mean because they represent centrality, but technically they’re slightly different. Mode refers to the most frequent value that appears in a data set, which may or may not be close to the mean. Median refers to the middle point of a data set, in other words 50% of the scores will fall under the median. For example, let’s assume the following 10 scores were collected during an experiment:
6 6 6 10 11 12 14 14 16 17
Mean = 11.2 The average of all scores (6+6+6+10+11+12+14+14+16+17 / 10)
Median = 11.5 Middle value (5 scores below and 5 scores above this value)
Mode = 6 Most frequent score
If the data set had an odd number of values, then the middle value is simply the median (ex. 1, 2, 3; 2 would be the median). Just remember there are slightly different ways to describe central tendency, but most often you’ll hear about the mean since mode and median are only reported for certain instances. When evaluating data based on calculated means it’s important to identify any outliers or extreme values in the data. Outliers and high variability of data can produce inflated or misleading results because the mean is sensitive to outliers and extreme values. In contrast, the median is not sensitive to outliers and extreme values, meaning the median won’t change if there is a greater spread in the data. If the mean is being reported it's important to also take note of the standard deviation to account for this.
Standard Deviation
The standard deviation is concerned with the variability or the spread of a data set. As previously mentioned, the mean is the central point of a data set and the standard deviation is an estimate of the variability around that central point. In other words, the standard deviation represents the typical amount that a score deviates from the mean. When the standard deviation is low that means the spread or dispersion of scores is small and more tightly grouped closer to the mean. When the standard deviation is large it signifies a widespread or high variability of scores, when this occurs the mean may not be a good representation of the data. The mean and standard deviation are forms of descriptive statistics which is useful for summarizing the data of a specific group. Meaning, they are only able to describe the data we have accrued, it cannot tell us if the results we acquired will happen again. Other statistical tests can fall under another form known as inferential statistics, which can allow (not always) for conclusions and generalizations of a sample to the larger population.
P-value
Probability is the underlying concept of p-values, which is the likelihood that something will occur. P-values reflect the level of significance, and the odds that the findings are due to chance, it’s impossible to have a p-value of 0 1. In exercise science the p-value is considered to be ‘significant’ at p < 0.05. Meaning, researchers believe that the odds of their findings occurring by chance are 5 in 100 or they are 95% sure the results were not by chance and the observed differences were a real change. In the results section when changes of a specific variable are reported there is a p-value reported after (e.g., 103.5 ± 15.1 ng/dL (p = 0.02)). In exercise and nutritional science, if the p-value is greater than 0.05 the result isn’t deemed to be significant. This is also stated as ‘supporting the null hypothesis’. The null hypothesis states there isn’t a relationship or difference and instead the findings are due to sampling error or random chance. Statistical tests are performed to either support or reject the null hypothesis and anything less than 0.05 rejects the null hypothesis and accepts the research hypothesis. Statistical significance is what you should identify when interpreting results, but significant differences aren’t the only thing you want to focus on. A study might show that one type of diet lost significantly more weight than another type of diet, but what if it was only by 0.5 lbs? That doesn’t mean much, but how do you determine if significant results are meaningful? While p-values provide statistical significance, effect sizes allow researchers to communicate practical significance of their results 3.
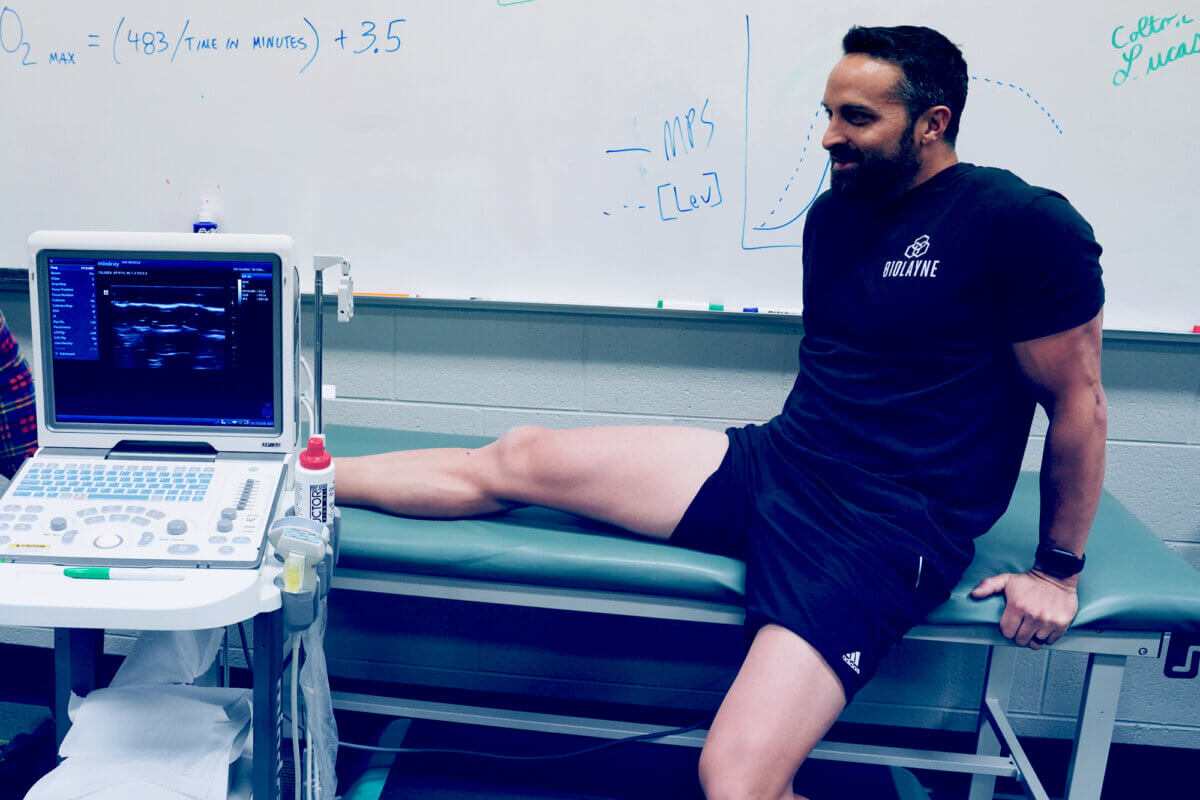