This article examines a study that employs innovative algorithms to analyze body weight fluctuations, providing insights that could transform our approach to weight loss. It highlights the significance of early weight trends as key indicators for long-term weight loss success.
Overview
What did they test? Based on data from a commercial weight loss program, the authors tested if an algorithm could identify key factors involved in weight loss.
What did they find? Body weight measures during the first two weeks of weight loss were able to classify individuals as having successful or unsuccessful weight loss over 12 months.
What does it mean for you? Early weight loss during a diet may be a good indicator to determine if a client or athlete is going to have a successful diet.
What’s the problem?
Weight loss poses a significant challenge for many people. The current obesity rate exceeds 40%, and over 70% of the U.S. population iis overweight 1. Even in research environments, where participants are not required to pay a coach or dietitian, and are often compensated for participating, the dropout rates from weight loss studies vary between 20-60%. This attrition occurs regardless of the diet type or intervention employed 2 3.
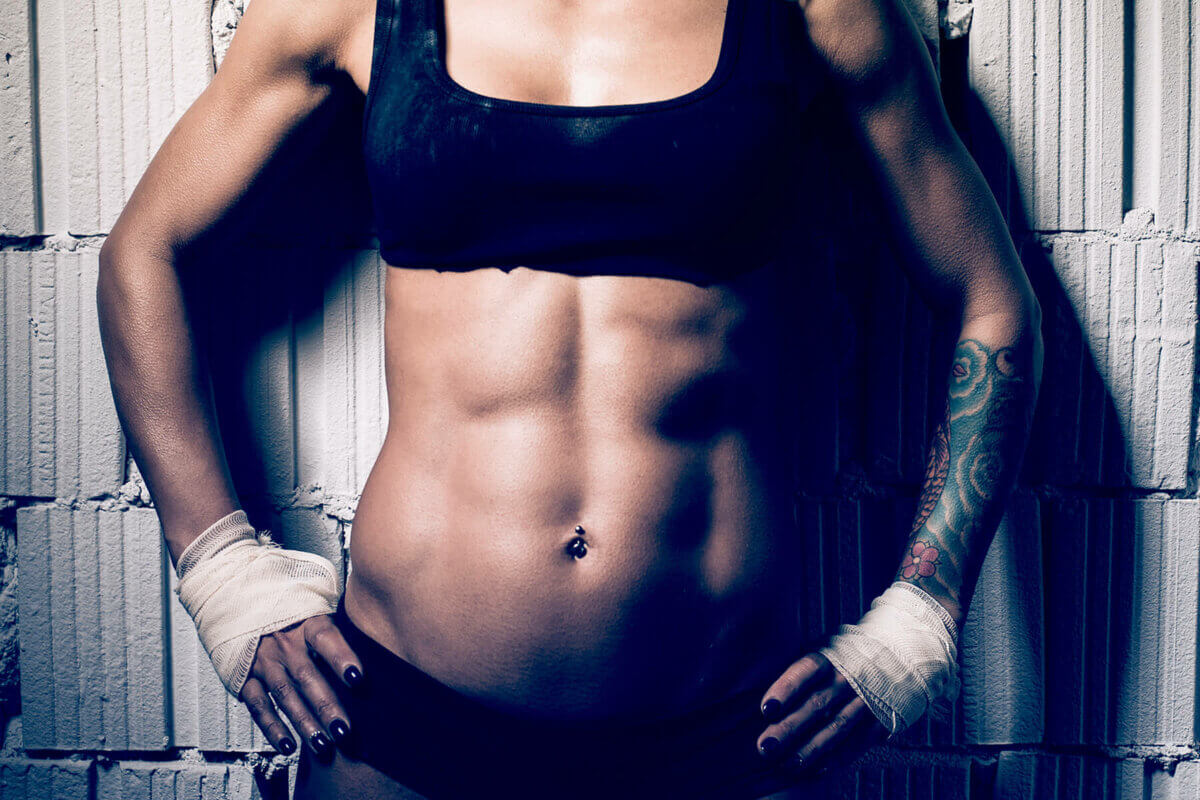
Previous research has suggested that initial weight loss can serve as a reliable indicator of long-term results. For instance, one study demonstrated that early positive responses to an mHealth treatment correlate with increased participation and a higher probability of attaining clinically significant weight loss 4. Another study highlighted that a weight loss of <2.0% early in a program significantly raises the risk of not achieving meaningful clinical weight loss by the program's conclusion 5.
Recent research increasingly utilizes trajectory modeling methods, such as latent class and growth mixture modeling, to sort individuals with overweight or obesity into distinct categories based on their patterns of weight loss 6 7 . This methodology holds promise for predicting long-term weight loss, taking into account the unique responses of individuals to various interventions. However, trajectory analyses commonly found in academic research may not directly apply to real-world settings like commercial or community weight management programs, which often feature varied program durations, irregular participation, and inconsistent weight tracking.
The authors of the current study focused on an online weight loss program to understand the predictive power of early weight loss, thereby potentially offering a more immediate metric for tailoring interventions to enhance long-term success in weight loss programs. The primary aim was to develop an algorithm to identify common patterns of weight change and classify participants’ weight trajectories with a method enabling accommodation of variable intervention duration and variable frequency of self- reporting, using data from participants enrolled in a commercial weight loss program.
Purpose
The purpose of this study was to develop an algorithm to identify common patterns of weight change and classify weight trajectories from participants who were in a commercial weight loss program for one year.